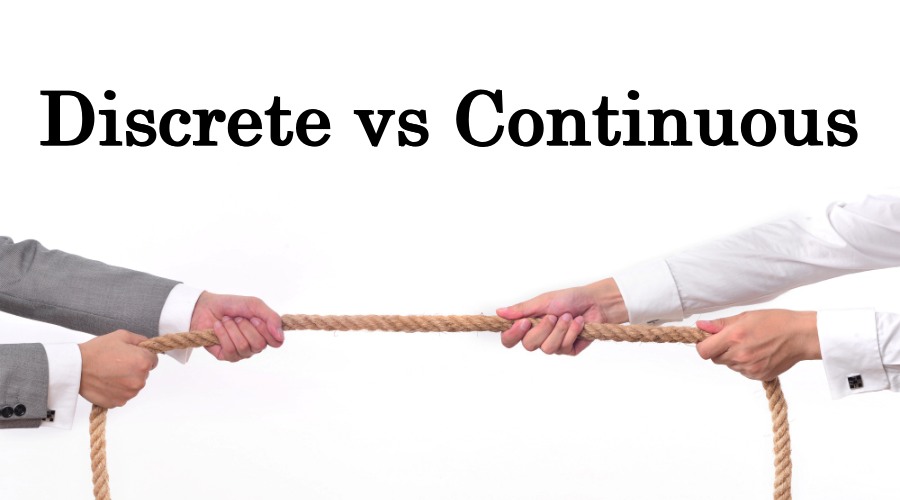
Discrete vs Continuous Data – What are the Key Differences?
Have you stumbled across the phrase “discrete vs continuous data” and want to get back into the statistical jungle with a quick but understandable answer?
Then you have found the machete here that frees you from all the stumbling blocks.
Because in this article, you will learn everything there is to know about discrete and continuous characteristics, which examples you can remember as a mnemonic, and which common mistakes you should avoid. This makes the next statistics exam or term paper an easy exercise.
What is a feature anyway?
Characteristics are properties that are examined in a survey. In a survey, an online survey form created with the help of a form builder apps, a feature corresponds to a question that was asked. Example of a characteristic is gender, year of birth, or marital status.
A distinction can be made here between qualitative and quantitative characteristics, which is usually expressed in numerical values (quantitative) and free text (qualitative).
A characteristic value is a concrete answer or the concrete value of a characteristic.
Frequency of characteristics
Before we can understand the terms discrete and continuous in connection with features, we must first look at when features are cumulative. This is always the case when the person with the characteristic, for example, the subject you are interviewing in a survey, can indicate several characteristics.
For example, the attribute “YouTube channels subscribed” can have the characteristics “shribe!”, “The Simple Club,” “Motivated Studies,” and “Cute Babies and Pets TV.”
The answer to the question “Which YouTube channels are you subscribed to?” can consist of none, one or more answers.
Characteristics are not cumulative if only one concrete answer is possible. For example, if the attribute is the date of birth, only one answer is possible, e.g., 01/01/1997.
Knowing the difference is important if you want to conduct a survey yourself and set up an online survey for this, for example. Here you also have to set the right conditions and answer options for each characteristic you want to examine so that the results also generate data that you can work with.
Discrete and continuous characteristics
If you now have an accumulative characteristic, in which several characteristics are possible, a distinction can be made between discrete and continuous characteristics.
A feature is discrete if only a limited number of answers or characteristics is possible.
It is also said here that the set of characteristic values can be counted. To the question, “Which of these European capitals have you already visited?” Again, none, one or more answers can be possible, but the answers are limited as there are only 48 European capitals.
A feature is continuous if an infinite number of answers or values are possible.
That will be the case if the question is: “How much time does it take you to do a 100m sprint?” Here the answer could be 11.7 seconds, but it could also be 12.754321 seconds. All you need is a fairly accurate gauge. But theoretically, it is possible.
Difference between discrete and continuous features and scale levels
If you already know my tutorial on the scale levels, then you will definitely notice something here. For the discrete features almost always appear to be on a nominal or ordinal scale.
The continuous features, on the other hand, are almost always found on a metric scale.
But only almost!
Because this is a common mistake that you have to watch out for in every statistics exam. Because just because you have a value with decimal places does not mean that you have a continuous characteristic or a metric scale here.
For example, a price in euros can be 2.37, i.e., 2 euros and 37 cents. But the value cannot be 2.375 euros, at least assuming that there is not half a cent (thanks to Wissenschafts Thurm for this example!)
By the way, a bitcoin has 8 possible decimal places, in case you were wondering or you have a very clever statistics professor with a penchant for trick questions.